You signed in with another tab or window. Reload to refresh your session.You signed out in another tab or window. Reload to refresh your session.You switched accounts on another tab or window. Reload to refresh your session.Dismiss alert
Found device 0 with properties:
name: GeForce GTX TITAN Z
major: 3 minor: 5 memoryClockRate (GHz) 0.8755
pciBusID 0000:84:00.0
Total memory: 5.94GiB
Free memory: 5.86GiB
2017-10-12 21:14:52.882773: I tensorflow/core/common_runtime/gpu/gpu_device.cc:961] DMA: 0
2017-10-12 21:14:52.882787: I tensorflow/core/common_runtime/gpu/gpu_device.cc:971] 0: Y
2017-10-12 21:14:52.882805: I tensorflow/core/common_runtime/gpu/gpu_device.cc:1030] Creating TensorFlow device (/gpu:0) -> (device: 0, name: GeForce GTX TITAN Z, pci bus id: 0000:84:00.0) Weights loaded!
2017-10-12 21:15:04.972817: I tensorflow/core/common_runtime/gpu/pool_allocator.cc:247] PoolAllocator: After 2024 get requests, put_count=1992 evicted_count=1000 eviction_rate=0.502008 and unsatisfied allocation rate=0.559289
2017-10-12 21:15:04.972892: I tensorflow/core/common_runtime/gpu/pool_allocator.cc:259] Raising pool_size_limit_ from 100 to 110
EP 0/100 | TRAIN_LOSS: 2.074034, 167.909124 | VALID_LOSS: 0.000000, 0.000000
EP 5/100 | TRAIN_LOSS: 1.574912, 165.120924 | VALID_LOSS: 0.000000, 0.000000
EP 10/100 | TRAIN_LOSS: 0.81238, 164.328942 | VALID_LOSS: 0.000000, 0.000000
.
.
.
EP 80/100 | TRAIN_LOSS: 0.24034, 26.891274 | VALID_LOSS: 0.000000, 0.000000
EP 85/100 | TRAIN_LOSS: 0.23912, 26.889122 | VALID_LOSS: 0.000000, 0.000000
EP 90/100 | TRAIN_LOSS: 0.23120, 26.871982 | VALID_LOSS: 0.000000, 0.000000
The valid loss is 0 because I do not calculate it (for timing purpose). Actually I calculated all the losses at the first time I ran the code and I saw that the valid loss decreased as low as the training loss.
When I test the model, it turns out that it always predict the same count (160) although the heat maps returned are different and quite closed to the label.
Could anyone please explain what is wrong with my code :D
The text was updated successfully, but these errors were encountered:
Hi, I used Keras to re-implement CountCeption model but got unexpected results and did not know how to fix it. Here is my model:
And here come the losses:
The valid loss is 0 because I do not calculate it (for timing purpose). Actually I calculated all the losses at the first time I ran the code and I saw that the valid loss decreased as low as the training loss.
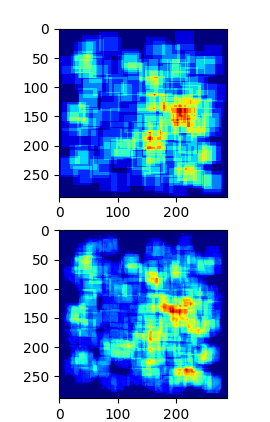
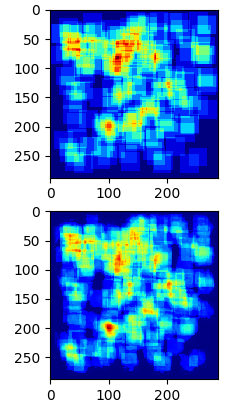
When I test the model, it turns out that it always predict the same count (160) although the heat maps returned are different and quite closed to the label.
Could anyone please explain what is wrong with my code :D
The text was updated successfully, but these errors were encountered: